Want to Know More About Youtube Digital Marketing Service?
페이지 정보

본문
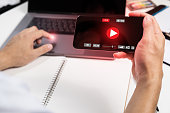
Historic Context and Current State
Over time, the formula advanced to incorporate extra advanced signals, including watch time, individual interaction, and customized checking out habits. The introduction of deep learning strategies even more boosted the algorithm's capability to forecast customer preferences and recommend pertinent web content.
Today, the YouTube algorithm runs on a multi-layered structure. The initial layer identifies a swimming pool of prospective video clips an individual may such as, while the 2nd layer rates these video clips based upon various aspects, consisting of user background, interaction metrics, and contextual signals. The effectiveness of this system is indisputable, yet it still encounters obstacles such as filter bubbles, material diversity, and the requirement for real-time adjustment to changing user preferences.
The Following Leap: Flexible Knowing Solutions
By evaluating individual interactions as they happen, the formula can fine-tune its predictions and provide more exact web content pointers. A customer might favor instructional material throughout weekdays and enjoyment on weekend breaks. Flexible understanding systems conquer this by making use of methods such as federated knowing, which allows the formula to find out from decentralized information sources without jeopardizing individual personal privacy.
: One of the criticisms of present referral systems is the creation of filter bubbles, where customers are repetitively shown similar content, limiting their exposure to diverse point of views.: The capability to adapt in real-time ensures that the formula continues to be pertinent also as user choices advance. By acknowledging arising patterns and individual passions in real-time, the formula can advertise new and relevant web content a lot more properly.
: YouTube presents adaptive learning systems in a phased rollout, beginning with a part of individuals in a certain region.: The rollout entails integrating real-time feedback loops, context-aware suggestions, and multi-modal information combination into the existing algorithm.: Evaluation reveals a 25% boost in the variety of material taken in by customers in the test team.
While the benefits of flexible learning systems are clear, their execution is not without obstacles.: Leveraging real-time customer information increases privacy worries.: Guaranteeing that the algorithm continues to be impartial and fair is a continuous challenge.
Future directions for study and development consist of exploring more innovative AI techniques, such as support discovering, to even more improve the flexibility and performance of suggestion systems. Additionally, incorporating customer comments more seamlessly right into the formula's knowing process can give important insights and boost its efficiency.
Final thought
The development of adaptive learning systems marks a substantial leap in YouTube video advertising service (patreon.com) formula optimization, using a more vibrant, context-aware, and individualized method to material recommendation. By resolving the constraints of existing algorithms and leveraging the current improvements in AI and ML, these systems assure to boost user involvement, web content diversity, and overall contentment. As YouTube remains to advance, the integration of adaptive understanding systems will unquestionably play a critical role fit the future of material exploration and referral.
These algorithms are essential to user involvement, content exploration, and system growth. The introduction of deep knowing techniques further boosted the algorithm's capacity to predict user preferences and recommend pertinent material.
The initial layer identifies a pool of prospective videos a customer could such as, while the 2nd layer ranks these videos based on many aspects, consisting of user background, interaction metrics, and contextual signals. By acknowledging arising trends and customer passions in real-time, the algorithm can advertise brand-new and appropriate web content extra effectively. By attending to the limitations of current algorithms and leveraging the most current innovations in AI and ML, these systems assure to enhance customer interaction, material variety, and overall complete satisfaction.
- 이전글8 Surefire Ways Sex Trafficking Will Drive What you are Marketing Into The Ground 24.08.09
- 다음글Answers about Laundry 24.08.09
댓글목록
등록된 댓글이 없습니다.